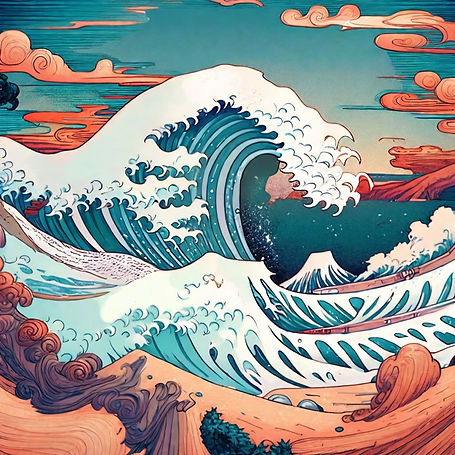

👨🏻💻 📊 🐶 🤖
Han Fang is currently a Senior Research Scientist Manager in GenAI at Meta, leading the applied research and engineering of Meta AI, the AI assistant with Llama 3 models - which is launched to Meta's family of apps and Smart Glasses.
Han's team is responsible for post-training of Meta AI’s production LLM, spanning SFT, RLHF, and Reward Models. He leads the development of Reinforcement Learning with User Feedback (RLUF) and the data flywheel, and the development the Orchestrator that empowers AI Agents with plugins, tool usage, and RAG.
Han holds a PhD in Applied Mathematics and Statistics and has published in top-tier conferences and journals with 5000+ citations. He is a recipient of the President’s Award to Distinguished Doctoral Students, the Woo-Jong Kim Dissertation Award, and the Excellence in Research Award. Han is a strong believer in giving back to the community. In his spare time, he serves as an industry advisory board member for University of Washington.
AI @ Meta

Meta AI on Llama3
Meta AI is our most advanced LLM-powered AI assistant. Built on Llama 3, our most advanced model to date, Meta AI is an intelligent assistant that is capable of complex reasoning, following instructions, visualizing ideas, and solving nuanced problems.
Now available within our family of apps and at meta.ai, you can learn more, imagine anything and get more things done.

Harmful content can evolve quickly. Our new AI system adapts to tackle it
We built and deployed Few-Shot Learner (FSL) that can adapt to new or evolving types of harmful content within days instead of months. It not only works in more than 100 languages, but also learns from different kinds of data, such as images and text, and it can strengthen existing AI models that are already deployed to detect other types of harmful content.

Training AI to detect hate speech in the real world
We’ve built and deployed an innovative system called Reinforcement Integrity Optimizer (RIO). RIO is an end-to-end optimized reinforcement learning (RL) framework. It’s now used to optimize hate speech classifiers that automatically review all content uploaded to Facebook and Instagram.

AI advances to better detect hate speech
We have a responsibility to keep the people on our platforms safe, and dealing with hate speech is one of the most complex and important components of this work. To better protect people, we have AI tools to quickly — and often proactively — detect this content.